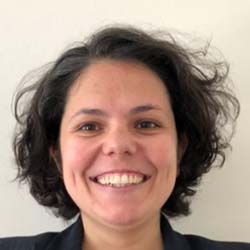
Iota Kaousar Nassr
Policy Analyst, OECD
The assessment of creditworthiness of prospective borrowers by banks and fintech lenders using AI and machine learning (ML) models is one of the most transformational use-cases of AI in finance.
Credit scoring models powered by AI provide risk scoring of thin-file clients with limited credit history or insufficient collateral, such as micro or young SMEs. Conventional credit information combined with big data (e.g. digital footprints) feeds into ML models enabling the extension of credit to unbanked/underbanked customers, potentially promoting financial inclusion.
Risks of bias and discrimination
This AI use-case, however, raises risks of disparate impact in credit outcomes and the potential for discriminatory or unfair lending, stemming from poor data management, combined with the lack of transparency or explainability of AI-based models. A neutral model that is trained with inadequate data, such as poorly labelled or inaccurate data or data that reflects underlying human prejudices, may produce inaccurate results even when fed with ‘good’ data and vice versa.
It is important to look at AI in finance as a technology that augments human capabilities, instead of replacing them.
Biased or discriminatory outcomes in AI credit rating models can be unintentional. Algorithms may combine facially neutral data points and treat them as proxies for immutable characteristics such as race or gender, thereby circumventing existing non-discrimination laws. While a credit officer may be careful not to include gender-based variants in a model, the model can infer the gender based on transaction activity and potentially use such knowledge in an assessment of creditworthiness.
The issue of explainability
Given the difficulty in comprehending, following or replicating the decision-making process of ML models, lenders may be limited in their ability to explain how credit decisions are made, while consumers may have little chance to understand what steps they should take to improve their ratings.
A decision-aid rather than a decision-maker
It is important to look at AI in finance as a technology that augments human capabilities, instead of replacing them. A combination of ‘human and machine’, whereby AI informs, rather than replaces, human judgment could allow for the benefits of AI to be realised, while maintaining the human safeguards of accountability and control in the ultimate decision.
Emphasis should be placed on human primacy in decision making for higher-value use-cases, such as lending, which have a significant impact on consumers. Clear communication and disclosures around the use of AI and the safeguards in place to protect consumers can help instil trust and confidence and promote the adoption of such innovative techniques in a safer manner.
Read more:
Artificial Intelligence, Machine Learning and Big Data in Finance: oecd.org/finance/Artificial-intelligence-machine-learning-big-data-in-finance.htm
OECD Business and Finance Outlook 2021: AI in Business and Finance: oecd.org/finance/oecd-business-and-finance-outlook-26172577.htm